The process of backtesting an AI stock prediction predictor is vital for evaluating the potential performance. This includes checking it against historical data. Here are ten tips on how to assess backtesting and ensure that the results are accurate.
1. In order to ensure adequate coverage of historical data it is crucial to have a reliable database.
Why is it important to test the model using a the full range of market data from the past.
How: Check that the period of backtesting includes diverse economic cycles (bull or bear markets, as well as flat markets) over multiple years. This lets the model be tested against a wide range of conditions and events.
2. Verify the real-time frequency of data and degree of granularity
The reason is that the frequency of data (e.g. every day, minute-by-minute) must be in line with the model's trading frequency.
How to build an high-frequency model, you need minute or tick data. Long-term models, however, can use daily or weekly data. A wrong degree of detail can provide misleading information.
3. Check for Forward-Looking Bias (Data Leakage)
The reason: When you use forecasts for the future based on data from the past, (data leakage), the performance of the system is artificially enhanced.
What to do: Confirm that the model only uses information available at every point in the backtest. Make sure that leakage is prevented by using safeguards such as rolling windows, or cross-validation that is based on time.
4. Determine performance beyond the return
The reason: Solely focussing on returns could be a distraction from other important risk factors.
How to use additional performance indicators such as Sharpe (risk adjusted return), maximum drawdowns, volatility and hit ratios (win/loss rates). This will give you a complete view of risk and the consistency.
5. Review the costs of transactions and slippage issues
Why is it important to consider trade costs and slippage could result in unrealistic profit targets.
What to do: Ensure that the backtest contains reasonable assumptions about spreads, commissions and slippage (the price movement between orders and their execution). The smallest of differences in costs could be significant and impact outcomes for models with high frequency.
6. Review Position Sizing and Risk Management Strategies
The reason: Proper sizing of positions and risk management impact both the risk exposure and returns.
How: Confirm the model's rules for positioning sizes are based on risk (like maximum drawsdowns or volatility targets). Backtesting should take into account diversification and risk-adjusted size, not only absolute returns.
7. Make sure that you have Cross-Validation and Out-of-Sample Testing
Why: Backtesting just on data from a small sample could result in an overfitting of the model, that is, when it performs well with historical data but not so well in real time.
It is possible to use k-fold Cross Validation or backtesting to assess the generalizability. Testing out-of-sample provides a clue for real-world performance when using unobserved data.
8. Examine the model's sensitivity to market conditions
The reason: The market's behavior varies significantly between flat, bull and bear phases which could affect model performance.
How to review back-testing results for different conditions in the market. A well-designed model will perform consistently, or should include adaptive strategies that can accommodate different regimes. Positive indicator Continuous performance in a range of environments.
9. Reinvestment and Compounding How do they affect you?
Reason: The strategy of reinvestment can result in overstated returns if they are compounded unintentionally.
What should you do: Examine whether the backtesting is based on real assumptions for compounding or investing such as only compounding the profits of a certain percentage or reinvesting profits. This will prevent inflated results due to exaggerated reinvestment strategies.
10. Verify the reliability of results from backtesting
Why: Reproducibility ensures that the results are reliable and not random or based on specific conditions.
What: Determine if the same data inputs can be utilized to replicate the backtesting process and generate identical results. The documentation should be able to generate the same results on different platforms or environments. This will give credibility to the backtesting process.
With these tips you can evaluate the backtesting results and gain a clearer idea of how an AI stock trade predictor could work. Read the most popular ai stocks hints for site tips including ai stock price, website for stock, best site to analyse stocks, stock investment, stocks for ai, ai in investing, ai to invest in, ai stock picker, open ai stock symbol, ai investing and more.
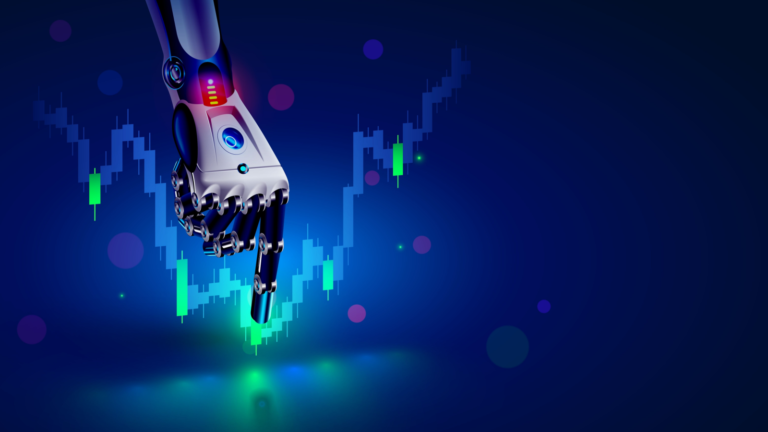
How To Use An Ai Prediction Of Trades In Stocks To Identify Meta Stock Index: 10 Best Strategies Here are ten tips for evaluating Meta stock with an AI model.
1. Understand Meta's business segments
What is the reason? Meta earns money in a variety of ways, such as through advertising on various platforms, including Facebook, Instagram, WhatsApp, and virtual reality, in addition to its virtual reality and metaverse projects.
Learn about the revenue contribution for each segment. Understanding growth drivers will help AI models make more accurate predictions of the future's performance.
2. Industry Trends and Competitive Analysis
The reason: Meta's performance is influenced by trends in digital marketing, social media usage, and competitors from other platforms such as TikTok and Twitter.
How: Ensure that the AI models are able to identify trends in the industry relevant to Meta, for example changes in user engagement and expenditures on advertising. Meta's market position and its possible challenges will be determined by a competitive analysis.
3. Earnings reports: How to determine their impact?
The reason: Earnings announcements can lead to significant movements in prices for stocks, particularly for firms that focus on growth, such as Meta.
How to use Meta's earnings calendar in order to monitor and evaluate historical earnings surprises. Include future guidance from Meta to evaluate the expectations of investors.
4. Utilize Technical Analysis Indicators
Why: Technical indicator can be used to detect patterns in the share price of Meta and possible reversal times.
How do you incorporate indicators like moving averages, Relative Strength Index (RSI) and Fibonacci Retracement levels into your AI model. These indicators help in identifying the most profitable places to enter and exit a trade.
5. Examine Macroeconomic Factors
Why? Economic conditions like inflation as well as interest rates and consumer spending could affect advertising revenue.
What should you do: Ensure that the model incorporates relevant macroeconomic indicators, such a GDP increase rate, unemployment rates as well as consumer satisfaction indices. This can improve a model's reliability.
6. Use Sentiment Analysis
What is the reason? Market sentiment can greatly influence stock prices especially in the tech sector, where public perception plays an important role.
How can you use sentiment analysis from social media, news articles and forums on the internet to determine the public's perception of Meta. This qualitative information can be used to give additional information about AI models and their predictions.
7. Monitor Regulatory & Legal Developments
What's the reason? Meta is subject to regulatory scrutiny in relation to privacy of data, antitrust concerns and content moderation which can impact its operations and stock performance.
How to keep up-to date on legal and regulatory changes that could affect Meta's business model. The model should take into consideration the potential risks that come with regulatory actions.
8. Testing historical data back to confirm it
What is the benefit of backtesting? Backtesting allows you to evaluate the performance of an AI model by comparing it to the past price changes or other significant events.
How to backtest predictions from models by using the historical Meta stock data. Compare the predictions to actual results, allowing you to gauge how accurate and robust your model is.
9. Review the Real-Time Execution Metrics
The reason is that efficient execution of trades is essential in maximizing Meta's price movements.
How to monitor execution metrics such as fill and slippage. Check the AI model's ability to predict the best entry and exit points for Meta trading in stocks.
Review Risk Management and Size of Position Strategies
How do you know: A good risk management strategy is crucial for protecting capital from volatile stocks such as Meta.
What should you do: Make sure the model includes strategies for position sizing and risk management based on Meta's stock volatility and the overall risk of your portfolio. This allows you to maximize your profits while minimizing potential losses.
By following these guidelines It is possible to examine the AI prediction of stock prices' ability to study and forecast Meta Platforms Inc.’s stock price movements, and ensure that they are precise and current in changing market conditions. View the most popular microsoft ai stock examples for website tips including top ai companies to invest in, artificial intelligence stock trading, trading stock market, ai investing, stocks and trading, ai stocks to invest in, good stock analysis websites, ai stock forecast, stock investment, stock trading and more.
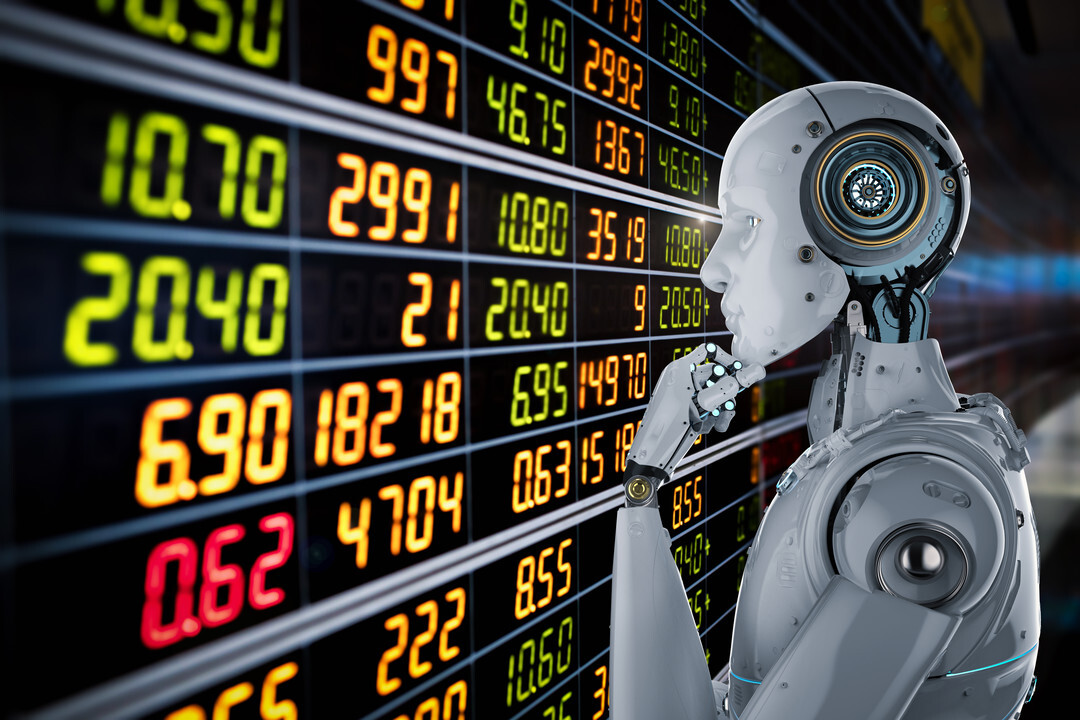